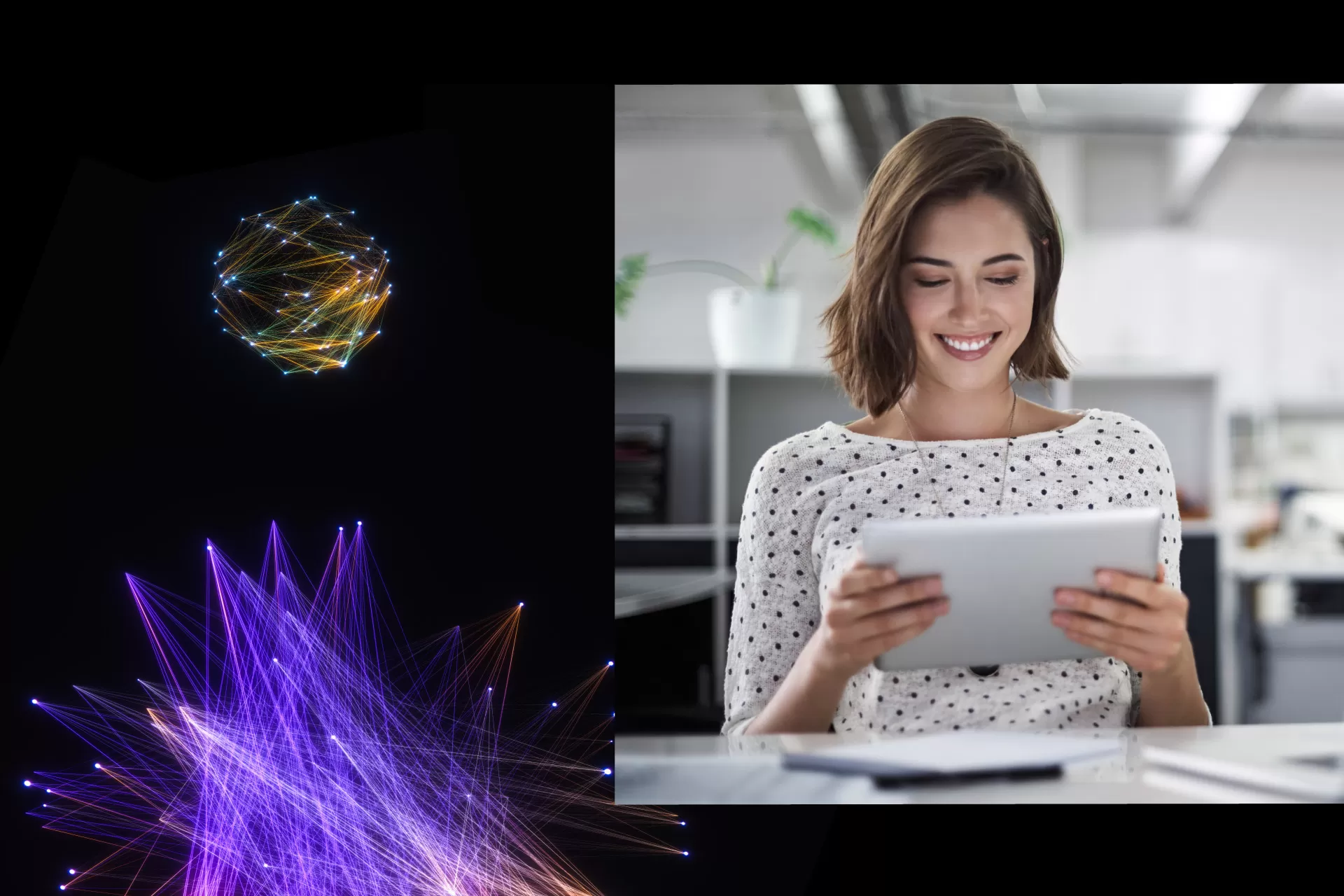
Generative AI: Exploring Its Depths and Possibilities
Open AI’s ChatGPT has taken the world by storm, just like the internet and the cloud did in the past. While generative AI has been around for some time, what sets ChatGPT apart from its predecessors is the simplicity of the platform that creates high-quality, responsive text in a matter of seconds.
What is Generative AI?
Generative AI is a powerful technology to generate new, realistic content using a set of algorithms created by large sets of training data. Generative AI mimics this behavior with way more data (almost the entire internet) and far more complex algorithms. The inputs and outputs for Generative AI are multimodal – text, audio, video, image, 3D images, and XR.
With the advent of generative adversarial networks, or GANs – a type of machine learning algorithm – in 2014, generative AI could create convincingly authentic synthetic content, improve efficiency, and introduce personalization and recommendation.
I know AI; how is Generative AI different?
Generative AI starts with a prompt that could be in the form of text, an image, a video, a design, musical notes, or any input that the AI system can process. The AI system recognizes the input and generates an output entirely on its own. It does not fetch answers from a database and does not return preformulated responses to queries. Traditional AI algorithms process existing or new data to give simple results.
Generative AI can therefore produce fresh content in the form of audio, visual, or deepfakes as opposed to traditional AI that specializes in honing and classifying data and detecting patterns and fraud. Both traditional and generative AI can be used for fraud detection, but the approach and outcomes are varied. Traditional AI relies on rule-based systems and anomaly detection methods based on which it flags outliers. Generative AI, once fed with training data comprising authentic and fraud data, identifies patterns of both behaviors and can create synthetic examples for both use cases that can be used to improve fraud detection accuracy.
Generative AI often uses neural network techniques such as transformers, GANs, and variational autoencoders (VAEs). Other kinds of AI, in contrast, use techniques including convolutional neural networks, recurrent neural networks, and reinforcement learning.
Generative AI use cases: The art of the possible
Industry | Summarization | Content generation | Personalization |
---|---|---|---|
Marketing and advertising |
|
|
|
High-tech manufacturing |
|
|
|
Customer service |
|
|
|
Insurance |
|
|
|
BFSI |
|
|
|
Art and entertainment |
|
|
|
eCommerce |
|
|
|
Healthcare |
|
|
|
The following tasks, among many others, can be done by generative AI:
- Generate campaign content and job descriptions
- Dialogue-based SMS support with no wait time
- Create a similar or variation of a content piece
- Summarize large pieces of content to develop a quicker understanding
- Sift through the knowledge base to get answers within an organization
- Human-like chatbot experiences
- Data entry and other repetitive jobs
- Monitor user sentiment
- Create code snippets and support development
- Basic QA for the code
Generative AI: At the forefront of digital transformation
Over the last decade, digital transformation was driven by –
- Widespread use of mobile phones in all spheres
- Advent of the cloud to enable data access from anywhere on the go
- IoT, which enabled feedback on customer usage analysis and continuous improvement
- Optimized and efficient operations through integrated systems
The four dimensions of digital transformation are customers, employees, products, and operations.
Customers: Generative AI can help businesses understand customers better and leverage its capabilities of summarization and personalization to offer customized solutions and improve customer support.
Employees: Employees can benefit from solutions such as knowledge base search and summarization to understand the processes and rationale behind decisions. Employee sentiment analysis and timely interventions from HR can improve employee satisfaction. Further, coding assistance and personalized L and D can propel employee growth.
Products: Content generation, concept simulation and validation, process and product optimization driven by the adoption of generative AI can drive product innovation.
Operations: Generative AI can recommend process improvements, supply chain optimization, query resolution, and summarization, which can result in better productivity.
Toward a brighter horizon with responsible generative AI
Generative AI brings forth a realm of possibilities that extend beyond our imagination, with immense potential to revolutionize multiple industries in unprecedented ways. As we embark on this journey, it becomes crucial to exercise caution and manage this technology responsibly, safeguarding society from potential risks.
At Zensar, we are committed to leading the charge in digital transformation while ensuring the safe and secure utilization of generative AI for our clients. By proactively identifying key risks, we have taken strategic measures to ensure the responsible use of this cutting-edge technology. Our AI Engineering Buddy solution, powered by Microsoft’s Azure suite, empowers us to drive design, code generation, summarization, and test case creation throughout the development lifecycle. With this approach, our goal is to fully unlock the potential of generative AI while prioritizing responsible and ethical practices.